· 4 min read
Understanding Deep Learning: a comprehensive overview
Deep learning represents a significant leap forward in the field of AI, enabling machines to learn and make decisions with unprecedented accuracy and complexity.
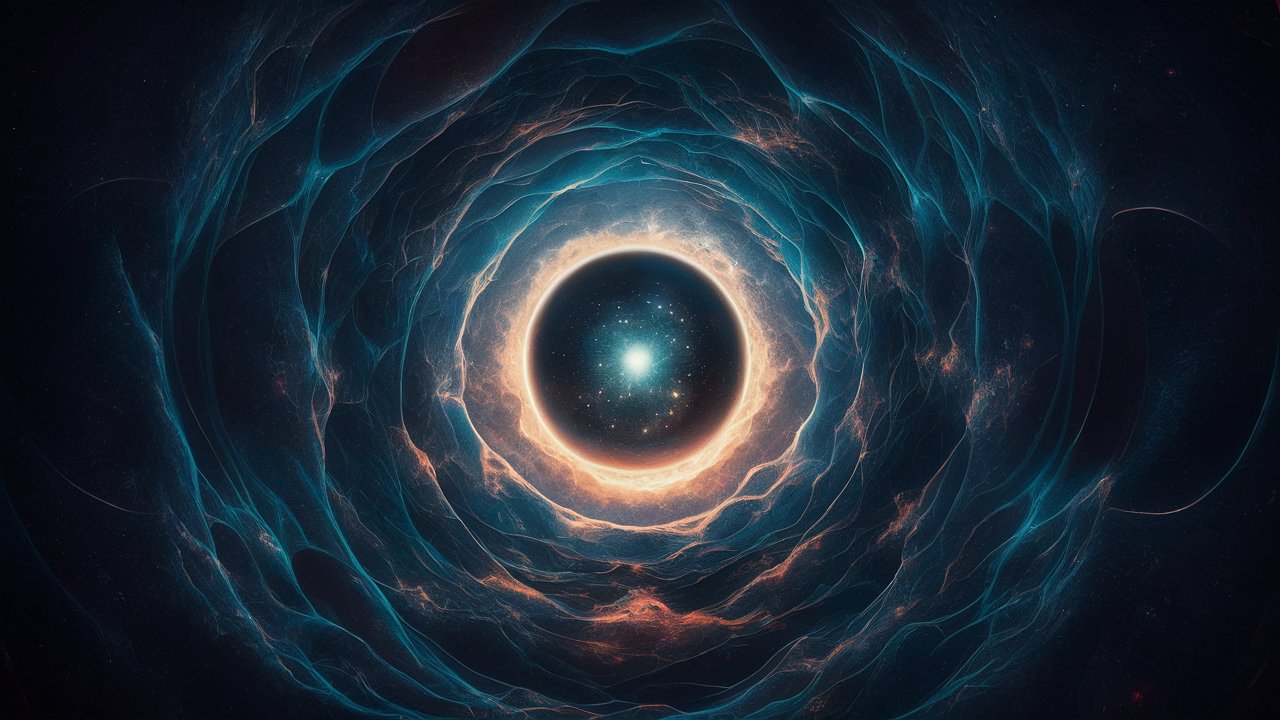
Deep learning is a fascinating and rapidly evolving subset of artificial intelligence (AI) that falls under the broader umbrella of machine learning. It involves the use of complex neural networks to recognize patterns, interpret data, and make predictions or decisions without explicit programming for each specific task. Let’s delve into the intricate world of deep learning, exploring its capabilities, methodologies, and applications.
The basics of Deep Learning
At its core, deep learning mimics the way the human brain operates through the use of artificial neural networks. These networks consist of layers of interconnected nodes (or neurons), each performing computations that contribute to the network’s overall ability to learn and process data. The term “deep” refers to the number of layers in the network - a deep neural network typically has multiple layers between the input and output, allowing it to model complex relationships and patterns in the data.
Deep Learning within the AI and Machine Learning spectrum
Deep learning is a subset of machine learning, which itself is a subset of AI. While traditional machine learning models rely on hand-crafted features and simpler algorithms, deep learning models automatically discover the representations needed for pattern recognition from raw data. This ability to learn directly from data without the need for manual feature extraction is what sets deep learning apart and makes it so powerful.
Recognizing complex patterns
One of the most remarkable capabilities of deep learning models is their ability to recognize complex patterns in various types of data. These models can analyze and interpret images, text, audio, and other forms of data to produce accurate insights and predictions. For example:
- Images: Convolutional Neural Networks (CNNs) are particularly adept at analyzing visual data. They can identify objects, faces, scenes, and even subtle patterns in medical images that may indicate disease.
- Text: Recurrent Neural Networks (RNNs) and Transformers, such as those used in models like GPT-3.5, excel at processing and generating human language, enabling tasks like translation, summarization, and sentiment analysis.
- Audio: Deep learning models can transcribe speech, recognize sounds, and even generate music, making them valuable in applications ranging from virtual assistants to automated customer service.
- Other Data Types: Beyond traditional data, deep learning models are being applied to sensory data such as temperature, touch, and even taste, enhancing fields like robotics and bioengineering.
The multimodal approach
Deep learning models are not limited to working with a single type of data. The multimodal approach leverages multiple forms of data, combining text, images, videos, sounds, and sensory inputs to create more comprehensive and accurate models. This methodology allows models to gain a more nuanced understanding of the world, leading to richer insights and more robust predictions.
For instance, a multimodal model might combine visual data from cameras with text data from documents to perform more accurate scene understanding. In medical diagnostics, combining imaging data with patient records can improve disease detection and treatment recommendations.
Embodied multimodal models
The concept of embodied multimodal models takes deep learning a step further by implementing these models in physical entities that can interact with the surrounding world. These embodied models are embedded in robots, smart devices, or other forms of casing, enabling them to gather and process diverse data in real-time.
For example, a robot equipped with cameras, microphones, and touch sensors can navigate and interact with its environment, learning from and responding to various stimuli. This embodiment allows for continuous data collection and adaptation, enhancing the model’s performance over time.
Applications and implications
The applications of deep learning are vast and growing. In healthcare, deep learning models assist in diagnosing diseases, personalizing treatment plans, and predicting patient outcomes. In autonomous driving, they enable vehicles to perceive and respond to their environment. In finance, they help detect fraud, optimize trading strategies, and provide personalized financial advice.
Moreover, deep learning is transforming entertainment, with advancements in video game AI, music generation, and content recommendation systems. It is also revolutionizing industries like agriculture, by improving crop monitoring and yield prediction, and environmental science, by enhancing climate modeling and disaster response.
Conclusion
Deep learning represents a significant leap forward in the field of AI, enabling machines to learn and make decisions with unprecedented accuracy and complexity. By recognizing patterns in diverse types of data, adopting multimodal approaches, and being embodied in interactive systems, deep learning models are pushing the boundaries of what machines can achieve. As research and technology continue to advance, the potential applications and benefits of deep learning are poised to expand even further, transforming industries and improving lives in ways we are only beginning to imagine.